Focus: Big data for infectious disease surveillance, modeling
January / February 2017 | Volume 16, Issue 1
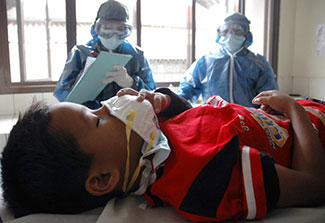
© 2006 Budi Yanto, Courtesy of Photoshare
Two nurses observe a young child suspected to have bird flu
in a hospital in Indonesia.
By Shana Potash
Big data derived from electronic health records, social media, the internet and other digital sources have the potential to provide more timely and detailed information on infectious disease threats or outbreaks than traditional surveillance methods, but there are challenges to overcome. A team of scientists led by the NIH reviewed the growing body of research on the subject and has published its analyses in a special issue of The Journal of Infectious Diseases.
Traditional infectious disease surveillance - typically based on laboratory tests and other epidemiological data collected by public health institutions - is the gold standard. But, the authors note it can include time lags, is expensive to produce, and typically lacks the local resolution needed for accurate monitoring. Further, it can be cost-prohibitive in low-income countries. In contrast, big data streams from internet queries, for example, are available in real time and can track disease activity locally, but have their own biases. Hybrid tools that combine traditional surveillance and big data sets may provide a way forward, the scientists suggest, serving to complement, rather than replace, existing methods.
"The ultimate goal is to be able to forecast the size, peak or trajectory of an outbreak weeks or months in advance in order to better respond to infectious disease threats. Integrating big data in surveillance is a first step toward this long-term goal," says Fogarty senior scientist Dr. Cecile Viboud, co-editor of the supplement. "Now that we have demonstrated proof of concept by comparing data sets in high-income countries, we can examine these models in low-resource settings where traditional surveillance is sparse."
Experts in epidemiology, computer science and modeling contributed to the 10-article supplement. The researchers report on the opportunities and challenges associated with three types of data: medical encounter files, such as records from health care facilities and insurance claim forms; crowdsourced data collected from volunteers who self-report symptoms in near real time (part of the "citizen science" movement); and data generated by the use of social media, the internet and mobile phones, which may include self-reporting of health, behavior and travel information.
But big data's potential must be tempered with caution, the authors say. Nontraditional data streams may lack key demographic identifiers such as age and sex, and the information they provide may underrepresent infants, children and the elderly, as well as residents of developing countries. Furthermore, social media outlets may not always be stable sources of data, as they can disappear if there is a loss of interest or financing. Most importantly, any novel data stream must be validated against established infectious disease surveillance data and systems, the authors emphasize.
The journal supplement, "Big Data for Infectious Disease Surveillance and Modeling," published in December 2016, contains reviews of recent uses of big data to strengthen disease surveillance, monitor antimicrobial resistance, identify adverse drug events, forecast disease outbreaks and connect travel patterns to the spread of disease.
Ensuring data privacy
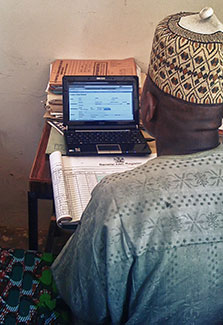
© 2010 eHealth Africa
Courtesy of Photoshare
A health worker in Nigeria enters data
into their Electronic Medical Record
System.
Big data offer a "tantalizing opportunity" to provide more information for public health surveillance, but the authors say its use for that purpose is decades behind other fields such as climatology and marketing. Electronic health records with identifying information removed, for example, may be a resource to monitor infectious diseases outcomes, vaccine uptake and adverse drug reactions. Applying the data to surveillance has been slow, the authors say, in part because of ethical concerns about patient privacy. There's also a scarcity of academic studies demonstrating how this type of data performs against traditional surveillance methods.
The supplement includes reviews of two nontraditional sources for monitoring influenza and other diseases - medical insurance claims and crowdsourced data.
Harvesting medical insurance claim data
Medical insurance claim forms, used in the U.S. and other countries, document the date and location of a doctor's office visit as well as a diagnosis code, which researchers say is useful in tracking disease outbreaks, especially in large populations. Working with anonymized claim form data made available for research, investigators found "excellent alignment" between claim data for flu-like illnesses and proven influenza activity reported by the CDC. The body of influenza research suggests medical claims data should be harvested to generate timely, local data on acute infections, according to the researchers.
Engaging the public to track the flu
A European surveillance system that began collecting crowdsourced data on influenza-like illnesses as part of a research project is now considered an adjunct to existing surveillance activities.
Called Influenzanet, the system uses standardized online surveys to gather information from volunteers who self-report their symptoms on a weekly basis. Data are analyzed in real time and national and regional results are posted on the website. Established in 2009, the tool is now being used by a number of European countries and is being expanded to collect information on Zika, salmonella and other diseases.
In their review, authors note the standardization of the technological and epidemiological framework makes it easier for countries to join Influenzanet and allows for coherent surveillance. The timeliness of the reporting and the inclusion of people who may not go to the doctor for treatment of the flu are other strengths.
Downsides include the potential for misreporting and the lack of validation by a physician or lab test. But the authors point out Influenzanet estimates of illness incidence compare well with data from traditional surveillance methods.
Aggregating antibiotic resistance data
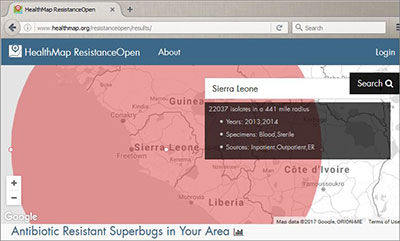
Courtesy of ResistanceOpen
The ResistanceOpen database, an online platform to monitor antimicrobial
resistance, is an extension of the HealthMap project.
Noting that antibiotic resistance is a growing concern around the world, U.S. and Canadian scientists developed an online platform to monitor it at the regional level. ResistanceOpen aggregates publicly available, online data from community health care institutions as well as regional, national and international bodies and displays the information on a navigable map. An analysis of the resource found that the online information compared favorably with traditional reporting systems in the U.S. and Canada.
The scientists who developed ResistanceOpen aim to expand the database and say the platform could help fill the gap in antimicrobial resistance surveillance in many low- and middle-income countries. ResistanceOpen is an extension of HealthMap, a project that collects and analyzes disparate online data sources to track infectious disease outbreaks around the world. HealthMap has been supported by private and public partners including the NIH, CDC and USAID.
Detecting adverse drug reactions
In addition to improving infectious disease surveillance, nontraditional data streams from the internet and social media have the potential to supplement traditional systems for reporting adverse drug reactions (ADRs). While consumers rarely use official ADR reporting systems, they do search the web for information about medications and share word of possible adverse reactions on social media sites and online health forums.
Mining and analyzing internet search logs and social media posts may detect ADR signals more quickly than traditional physician-based reporting systems, but there are challenges. One of the many ethical questions surrounding the use of these nontraditional sources is whether privately held data should be accessible for public health research.
Comparing epidemic and weather forecasting
In a comparison of the relatively new field of epidemic forecasting to the better-established one of weather forecasting, the authors note the former is much more difficult given that there is less observational data for disease, and because human behavior has the potential to rapidly alter the course of an epidemic.
Internet data streams, such as search queries and social media posts, may aid epidemic forecasting by providing information in near real time and at a more local level. But internet data, the authors say, are less reliable than information collected from weather stations and the availability can vary because of limited internet access in many developing countries.
Harnessing spatial big data
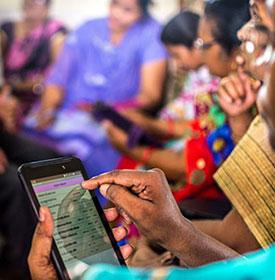
© 2015 Girdhari Bora/IntraHealth International
Courtesy of Photoshare
A woman uses a smart phone in India.
To determine where an outbreak originated or where future ones may occur, for example, epidemiologists need spatial data. Medical insurance claims, social media posts and mobile phones have the potential to fill geographical information gaps. But, the authors point out, there are technical, practical and ethical issues that must be addressed. They note possible solutions to protect privacy, such as masking individual-level information by aggregating collected data to larger spatial resolutions.
Connecting mobility to infectious diseases
With appropriate safeguards to ensure anonymity, call data records from mobile phones may provide researchers "an unprecedented opportunity" to determine how travel affects disease transmission. Studies of malaria and rubella in Kenya showed how call data improved the understanding of the spatial transmission of those diseases. Because mobile phone data has biases young children are not likely to be represented, for example. The authors say more research is needed to determine if mobility patterns derived from call data records are representative of general travel patterns.
Culling information from internet reports
Online news articles and health bulletins from public health agencies can also be manually dissected to model the sequence of transmission chains in an outbreak. The transmission dynamics and risk factors of the Ebola epidemic in West Africa and a Middle East Respiratory Syndrome outbreak in South Korea were elucidated by this approach. Internet findings were in line with traditional data, providing a proof of concept that this approach can be generalized and automated to a variety of online sources and generate information on disease transmission. This is particularly useful to improve situational awareness and guide public health interventions during emerging infectious disease crises, when traditional surveillance data are particularly scarce.
Managing epidemic simulation data
Researchers also describe the benefits of a novel, publicly available epidemic simulation data management system, called epiDMS, which provides storage and indexing services for large data simulation sets, as well as search functionality and data analysis to aid decision makers during health care emergencies.
While the new hybrid models that combine traditional and digital disease surveillance methods show promise, the scientists agree there is still an overall scarcity of reliable surveillance information, especially compared to other fields such as climatology, where the data sets are huge.
"To be able to produce accurate forecasts, we need better observational data that we just don't have in infectious diseases," notes Dr. Shweta Bansal of Georgetown University, a co-editor of the supplement. "There's a magnitude of difference between what we need and what we have, so our hope is that big data will help us fill this gap."
Multidisciplinary initiatives such as the NIH-led Big Data to Knowledge (BD2K) program will be instrumental in expanding the use of big data in research, as noted in the supplement.
The publication's authors include scientists affiliated with Fogarty's Research and Policy for Infectious Disease Dynamics (RAPIDD) program, grantees from NIH's National Institute of General Medical Sciences (NIGMS), and researchers from nearly 20 universities throughout North America and Europe.
The supplement was produced with support from Fogarty and Georgia State, Northeastern and Georgetown universities.
More Information
To view Adobe PDF files,
download current, free accessible plug-ins from Adobe's website.